Documents
Poster
Insights into End-to-End Learning Scheme for Language Identification
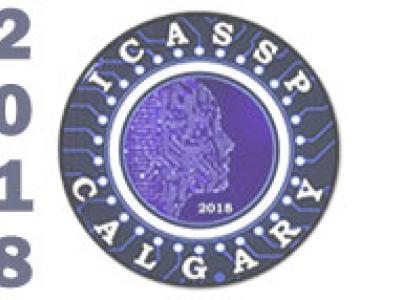
- Citation Author(s):
- Submitted by:
- Weicheng Cai
- Last updated:
- 13 April 2018 - 9:32am
- Document Type:
- Poster
- Document Year:
- 2018
- Event:
- Presenters:
- Weicheng Cai
- Paper Code:
- 3855
- Categories:
- Log in to post comments
A novel interpretable end-to-end learning scheme for language identification is proposed. It is in line with the classical GMM i-vector methods both theoretically and practically. In the end-to-end pipeline, a general encoding layer is employed on top of the front-end CNN, so that it can encode the variable-length input sequence into an utterance level vector automatically. After comparing with the state-of-the-art GMM i-vector methods, we give insights into CNN, and reveal its role and effect in the whole pipeline. We further introduce a general encoding layer, illustrating the reason why they might be appropriate for language identification. We elaborate on several typical encoding layers, including a temporal average pooling layer, a recurrent encoding layer and a novel learnable dictionary encoding layer. We conducted experiment on NIST LRE07 closed-set task, and the results show that our proposed end-to-end systems achieve state-of-the-art performance.