Documents
Presentation Slides
INSTANT ADAPTIVE LEARNING: AN ADAPTIVE FILTER BASED FAST LEARNING MODEL CONSTRUCTION FOR SENSOR SIGNAL TIME SERIES CLASSIFICATION ON EDGE DEVICES
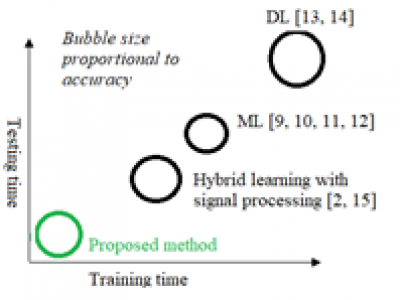
- Citation Author(s):
- Submitted by:
- Arijit Ukil
- Last updated:
- 14 May 2020 - 12:56pm
- Document Type:
- Presentation Slides
- Document Year:
- 2020
- Event:
- Presenters:
- Arijit Ukil
- Paper Code:
- 3324
- Categories:
- Log in to post comments
Construction of learning model under computational and energy constraints, particularly in highly limited training time requirement is a critical as well as unique necessity of many practical IoT applications that use time series sensor signal analytics for edge devices. Yet, majority of the state-of-the-art algorithms and solutions attempt to achieve high performance objective (like test accuracy) irrespective of the computational constraints of real-life applications. In this paper, we propose Instant Adaptive Learning that characterizes the intrinsic signal processing properties of time series sensor signals using linear adaptive filtering and derivative spectrum to efficiently construct a low-cost learning model followed by standard classification algorithms. Our empirical studies on a number of time series sensor signals from publicly available time series database (UCR) demonstrate that with slight trade-off in performance, the proposed method achieves very fast learning capability.