Documents
Poster
Inter- and Intra- Patient ECG Heartbeat Classification For Arrhythmia Detection: a Sequence to Sequence Deep Learning Approach
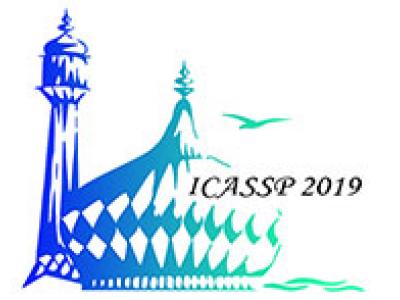
- Citation Author(s):
- Submitted by:
- Sajad Mousavi
- Last updated:
- 7 May 2019 - 3:03pm
- Document Type:
- Poster
- Document Year:
- 2019
- Event:
- Paper Code:
- 3824
- Categories:
- Keywords:
- Log in to post comments
Electrocardiogram (ECG) signal is a common and powerful tool to study heart function and diagnose several abnormal arrhythmias. While there have been remarkable improvements in cardiac arrhythmia classification methods, they still cannot offer acceptable performance in detecting different heart conditions, especially when dealing with imbalanced datasets. In this paper, we propose a solution to address this limitation of current classification approaches by developing an automatic heartbeat classification method using deep convolutional neural networks and sequence to sequence models. We evaluated the proposed method on the MIT-BIH arrhythmia database, considering the intra-patient and inter-patient paradigms, and the AAMI EC57 standard. The evaluation results for both paradigms show that our method achieves the best performance in the literature (a positive predictive value of 96.46% and sensitivity of 100% for the category S, and a positive predictive value of 98.68% and sensitivity of 97.40% for the category F for the intra-patient scheme; a positive predictive value of 92.57% and sensitivity of 88.94% for the category S, and a positive predictive value of 99.50% and sensitivity of 99.94% for the category V for the inter-patient scheme.). The source code is available at https://github.com/SajadMo/ECG-Heartbeat-Classification-seq2seq-model.
https://ieeexplore.ieee.org/abstract/document/8683140