Documents
Presentation Slides
Interpretable Learned Image Compression: A Frequency Transform Decomposition Perspective
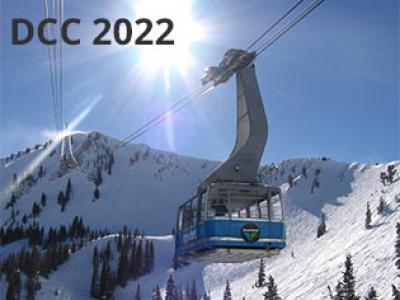
- Citation Author(s):
- Submitted by:
- Yuefeng Zhang
- Last updated:
- 3 March 2022 - 8:58am
- Document Type:
- Presentation Slides
- Document Year:
- 2022
- Event:
- Presenters:
- Yuefeng Zhang
- Paper Code:
- 191
- Categories:
- Log in to post comments
Image compression is a key problem in this age of information explosion. With the help of machine learning, recent studies have shown that learning-based image compression methods tend to surpass traditional codecs. Image compression can be split into three steps: transform, quantization, and entropy estimation.
However, the transform step in traditional codecs lacks flexibility because of the strict mathematical premise while the transform in most learning-based codecs neglects its intrinsic interpretation. After observing compression degradation degree varies on different frequency bands, we propose an end-to-end compression model from the frequency perspective with a frequency-pyramid transform and a frequency-aware fusion module. Intuitively, we can infer that the low-frequency part contains the global structure while the high-frequency part gets finer details, satisfying the feature of human visual system (HVS). In our proposed model, independent probability estimation models are set for each frequency split. Extensive experiments are conducted to demonstrate that our model outperforms all traditional codecs (e.g., JPEG, JPEG2000, HEVC, and VVC) on MS-SSIM metric on both Kodak and CLIC2020 professional test datasets. Taking BPG-4:4:4 as the anchor, our proposed model achieves 11.6% BD-rate reduction under PSNR measurement, which is evaluated on the Kodak dataset.
Comments
Nice to be here!!!
Welcome to contact us if you have any questions & suggestions!