Documents
Poster
INVERTIBLE VOICE CONVERSION WITH PARALLEL DATA
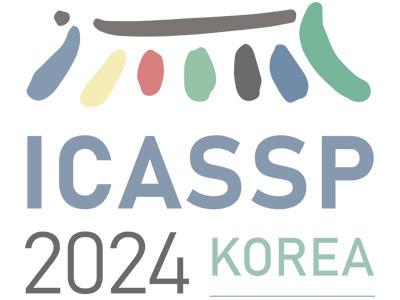
- Citation Author(s):
- Submitted by:
- Zexin Cai
- Last updated:
- 28 March 2024 - 5:12pm
- Document Type:
- Poster
- Document Year:
- 2024
- Event:
- Presenters:
- Zexin Cai
- Paper Code:
- SLP-P5.1
- Categories:
- Log in to post comments
This paper introduces an innovative deep learning framework for parallel voice conversion to mitigate inherent risks associated with such systems. Our approach focuses on developing an invertible model capable of countering potential spoofing threats. Specifically, we present a conversion model that allows for the retrieval of source voices, thereby facilitating the identification of the source speaker. This framework is constructed using a series of invertible modules composed of affine coupling layers to ensure the reversibility of the conversion process. We conduct comprehensive training and evaluation of the proposed framework using parallel training data. Our experimental results reveal that this approach achieves comparable performance to non-invertible systems in voice conversion tasks. Notably, the converted outputs can be seamlessly reverted to the original source inputs using the same parameters employed during the forwarding process. This advancement holds considerable promise for elevating the security and reliability of voice conversion.