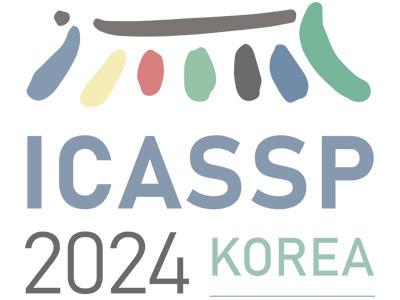
- Read more about Self-supervised Speaker Verification Employing a Novel Clustering Algorithm
- Log in to post comments
Clustering is an unsupervised learning technique, which leverages a large amount of unlabeled data to learn cluster-wise representations from speech. One of the most popular self-supervised techniques to train a speaker verification system is to predict the pseudo-labels using clustering algorithms and then train the speaker embedding network using the generated pseudo-labels in a discriminative manner. Therefore, pseudo-labels - driven self-supervised speaker verification systems' performance relies heavily on the accuracy of the adopted clustering algorithms.
- Categories:
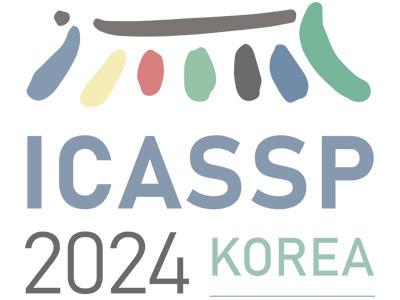
- Read more about ”IT IS OKAY TO BE UNCOMMON”: QUANTIZING SOUND EVENT DETECTION NETWORKS ON HARDWARE ACCELERATORS WITH UNCOMMON SUB-BYTE SUPPORT
- Log in to post comments
If our noise-canceling headphones can understand our audio environments, they can then inform us of important sound events, tune equalization based on the types of content we listen to, and dynamically adjust noise cancellation parameters based on audio scenes to further reduce distraction. However, running multiple audio understanding models on headphones with a limited energy budget and on-chip memory remains a challenging task.
- Categories:
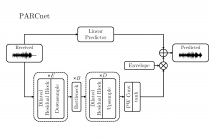
- Read more about Hybrid Packet Loss Concealment for Real-Time Networked Music Applications
- Log in to post comments
Real-time audio communications over IP have become essential to our daily lives. Packet-switched networks, however, are inherently prone to jitter and data losses, thus creating a strong need for effective packet loss concealment (PLC) techniques.
- Categories:
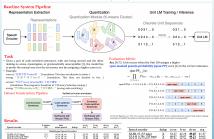
- Read more about Zero Resource Code-switched Speech Benchmark Using Speech Utterance Pairs For Multiple Spoken Languages
- Log in to post comments
We introduce a new zero resource code-switched speech benchmark designed to directly assess the code-switching capabilities of self-supervised speech encoders. We showcase a baseline system of language modeling on discrete units to demonstrate how the code-switching abilities of speech encoders can be assessed in a zero-resource manner. Our experiments encompass a variety of well-known speech encoders, including Wav2vec 2.0, HuBERT, XLSR, etc. We examine the impact of pre-training languages and model size on benchmark performance.
- Categories:
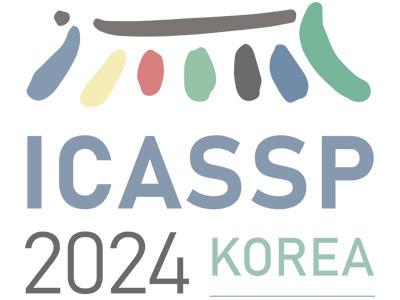
- Read more about TF-SepNet: An Efficient 1D Kernel Design in CNNs for Low-Complexity Acoustic Scene Classification
- Log in to post comments
Recent studies focus on developing efficient systems for acoustic scene classification (ASC) using convolutional neural networks (CNNs), which typically consist of consecutive kernels. This paper highlights the benefits of using separate kernels as a more powerful and efficient design approach in ASC tasks. Inspired by the time-frequency nature of audio signals, we propose TF-SepNet, a CNN architecture that separates the feature processing along the time and frequency dimensions. Features resulted from the separate paths are then merged by channels and directly forwarded to the classifier.
- Categories:
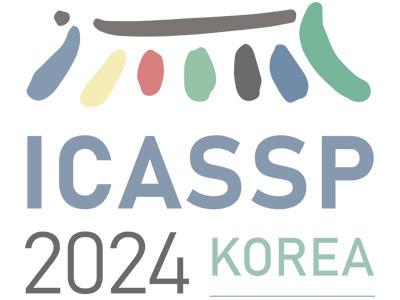
- Read more about INVERTIBLE VOICE CONVERSION WITH PARALLEL DATA
- Log in to post comments
This paper introduces an innovative deep learning framework for parallel voice conversion to mitigate inherent risks associated with such systems. Our approach focuses on developing an invertible model capable of countering potential spoofing threats. Specifically, we present a conversion model that allows for the retrieval of source voices, thereby facilitating the identification of the source speaker. This framework is constructed using a series of invertible modules composed of affine coupling layers to ensure the reversibility of the conversion process.
- Categories:
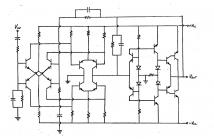
___Although dated, this student thesis is re-published as the proposed negative feedback topology and the current mode arrangement of silicon bipolar junction transistors is rarely elaborated in the many excellent contemporary books on audio power amplifier design.
- Categories:
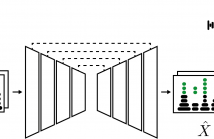
- Categories:
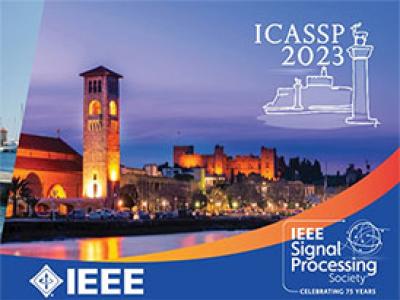
- Read more about Efficient Large-scale Audio Tagging via Transformer-to-CNN Knowledge Distillation
- Log in to post comments
Audio Spectrogram Transformer models rule the field of Audio Tagging, outrunning previously dominating Convolutional Neural Networks (CNNs). Their superiority is based on the ability to scale up and exploit large-scale datasets such as AudioSet. However, Transformers are demanding in terms of model size and computational requirements compared to CNNs. We propose a training procedure for efficient CNNs based on offline Knowledge Distillation (KD) from high-performing yet complex transformers.
- Categories:
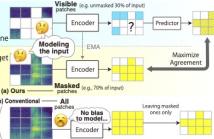
- Read more about MASKED MODELING DUO: LEARNING REPRESENTATIONS BY ENCOURAGING BOTH NETWORKS TO MODEL THE INPUT
- Log in to post comments
Masked Autoencoders is a simple yet powerful self-supervised learning method. However, it learns representations indirectly by reconstructing masked input patches. Several methods learn representations directly by predicting representations of masked patches; however, we think using all patches to encode training signal representations is suboptimal. We propose a new method, Masked Modeling Duo (M2D), that learns representations directly while obtaining training signals using only masked patches.
- Categories: