Documents
Poster
INVESTIGATING THE CLUSTERS DISCOVERED BY PRE-TRAINED AV-HUBERT
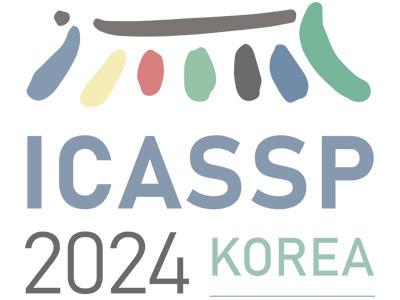
- DOI:
- 10.60864/84de-5162
- Citation Author(s):
- Submitted by:
- Tamas Grosz
- Last updated:
- 6 June 2024 - 10:27am
- Document Type:
- Poster
- Document Year:
- 2024
- Event:
- Presenters:
- Tamas Grosz
- Paper Code:
- SLP-P29.7
- Categories:
- Log in to post comments
Self-supervised models, such as HuBERT and its audio-visual version AV-HuBERT, have demonstrated excellent performance on various tasks. The main factor for their success is the pre-training procedure, which requires only raw data without human transcription. During the self-supervised pre-training phase, HuBERT is trained to discover latent clusters in the training data, but these clusters are discarded, and only the last hidden layer is used by the conventional finetuning step. We investigate what latent information the AV-HuBERT model managed to uncover via its clusters and can we use them directly for speech recognition. To achieve this, we consider the sequence of cluster ids as a 'language' developed by the AV-HuBERT and attempt to translate it to English text via small LSTM-based models. These translation models enable us to investigate the relations between the clusters and the English alphabet, shedding light on groups of latent clusters specialized to recognise specific phonetic groups. Our results demonstrate that using the pre-trained system as a quantizer, we are able to compress the video to as low as 275 bit/sec while maintaining acceptable speech recognition accuracy. Furthermore, compared to the conventional finetuning step, our solution has considerably lower computational cost.