Documents
Presentation Slides
INVESTIGATION OF ROBUSTNESS OF HUBERT FEATURES FROM DIFFERENT LAYERS TO DOMAIN, ACCENT AND LANGUAGE VARIATIONS
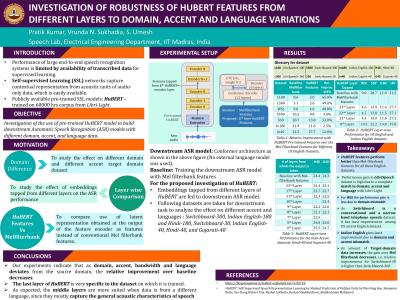
- Citation Author(s):
- Submitted by:
- Pratik Kumar
- Last updated:
- 9 May 2022 - 11:06am
- Document Type:
- Presentation Slides
- Document Year:
- 2022
- Event:
- Presenters:
- Pratik Kumar
- Paper Code:
- 5182
- Categories:
- Log in to post comments
In this paper, we investigate the use of pre-trained HuBERT model to build downstream Automatic Speech Recognition (ASR) models using data that have differences in domain, accent and even language. We use the standard ESPnet recipe with HuBERT as pretrained models whose output is fed as input features to a downstream Conformer model built from target domain data. We compare the performance of HuBERT pre-trained features with the baseline Conformer model built with Mel-filterbank features. We observe that as the domain, accent and bandwidth (as in the case of Switchboard data) vary, the relative improvements in performance over baseline decrease significantly. Further, with more labelled data in the target domain, the relative improvement narrows down, and both systems become comparable. We also investigate the effect on ASR performance when output from intermediate layers of HuBERT are used as features and show that these are more suitable for data in a different language, since they capture more of the acoustic representation. Finally, we compare the output from Convolutional Neural Network (CNN) Feature encoder used in pre-trained models with the Mel-filterbank features and show that Mel-filterbanks are often better features for modelling data from different domains.