Documents
Presentation Slides
ITERATIVE SUBNETWORK WITH LINEAR HIERARCHICAL ORDERING FOR HUMAN POSE ESTIMATION
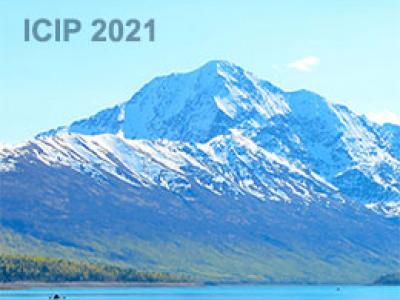
- Citation Author(s):
- Submitted by:
- Shek Wai Chu
- Last updated:
- 26 September 2021 - 10:01pm
- Document Type:
- Presentation Slides
- Document Year:
- 2021
- Event:
- Presenters:
- SHEK WAI CHU
- Paper Code:
- MLR-APPL-IVSMR-3.6
- Categories:
- Log in to post comments
Human pose estimation is a long-standing and challenging problem in computer vision. Many recent advancements in the field have relied on complex structure refinement and specific human joint graphical relations. However, progress has been saturated in terms of accuracy. Each time, new state-of-the-art approaches only improve accuracy by less than 0.3% in the MPII test set despite using complicated model structures. Most recent developments can be summarized into two main ideas: 1) refinement subnetwork to improve predictions iteratively and 2) exploitation of human joint graphical relations. In this work, we present how efficient and simple
iterative subnetworks with linear hierarchical ordering based on the aforementioned ideas can help to improve accuracy on strong backbone models. Different versions of iterative subnetwork
are examined. Significant improvements on difficult body part predictions such as wrists and ankles using a simple convolution subnetwork are observed. Further improvements can be made by using a large receptive field subnetwork such as axial-transformer.