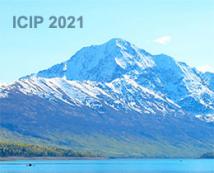
ICIP 2021 - The International Conference on Image Processing (ICIP), sponsored by the IEEE Signal Processing Society, is the premier forum for the presentation of technological advances and research results in the fields of theoretical, experimental, and applied image and video processing. ICIP has been held annually since 1994, brings together leading engineers and scientists in image and video processing from around the world. Visit website.
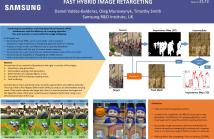
- Read more about FAST HYBRID IMAGE RETARGETING
- Log in to post comments
Image retargeting changes the aspect ratio of images while aiming to preserve content and minimise noticeable distortion. Fast and high-quality methods are particularly relevant at present, due to the large variety of image and display aspect ratios. We propose a retargeting method that quantifies and limits warping distortions with the use of content-aware cropping. The pipeline of the proposed approach consists of the following steps. First, an importance map of a source image is generated using deep semantic segmentation and saliency detection models.
- Categories:
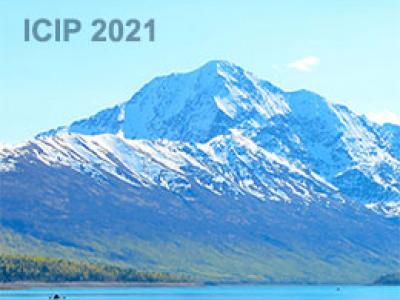
- Read more about Semi-Supervised Object Detection with Sparsely Annotated Dataset
- Log in to post comments
When training an anchor-based object detector with a sparsely annotated dataset, the effort required to locate positive examples can cause performance degradation. Because anchor-based object detection models collect positive examples under IoU between anchors and ground-truth bounding boxes, in a sparsely annotated image, some objects that are not annotated can be assigned as negative examples, such as backgrounds.
- Categories:
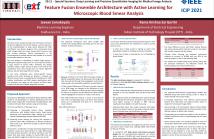
- Read more about Feature Fusion Ensemble Architecture With Active Learning For Microscopic Blood Smear Analysis
- Log in to post comments
The blood smear analysis provides vital information and forms the basis to diagnose most of the diseases. With recent developments, deep learning methods can analyze the microscopic blood sample using image processing and classification tasks with less human effort and increased accuracy.
- Categories:
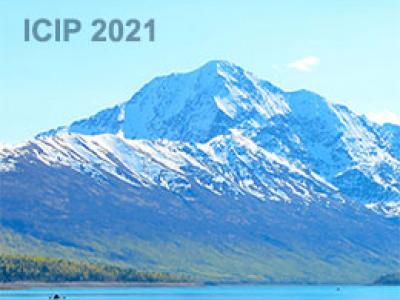
- Read more about A Hybrid Two stream Approach For Multi Person Action Recognition in Top view 360 degree Videos
- Log in to post comments
Action recognition in top-view 360° videos is an emerging research topic in computer vision. Existing work utilizes a global projection method to transform 360° video frames to panorama frames for further processing. However, this unwrapping suffers from a problem of geometric distortion i.e., people present near the centre in the 360° video frames appear highly stretched and distorted in the corresponding panorama frames (observed in 37.5% of the total panorama frames in 360Action dataset).
posterv6.pdf

video_pptv3.pdf

- Categories:
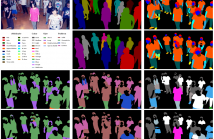
- Read more about Describe me if you can! Characterized instance-level human parsing
- Log in to post comments
Several computer vision applications such as person search or online fashion rely on human description. The use of instance-level human parsing (HP) is therefore relevant since it localizes semantic attributes and body parts within a person. But how to characterize these attributes? To our knowledge, only some single-HP datasets describe attributes with some color, size and/or pattern characteristics. There is a lack of dataset for multi-HP in the wild with such characteristics.
- Categories:
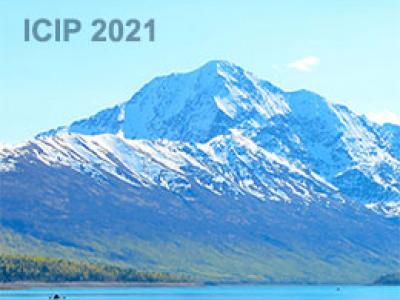
- Categories:
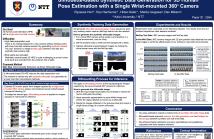
- Read more about Silhouette-based Synthetic Data Generation for 3D Human Pose Estimation with a Single Wrist-mounted 360° Camera
- Log in to post comments
In this paper, we propose a framework for 3D human pose estimation with a single 360° camera mounted on the user's wrist. Perceiving a 3D human pose with such a simple setting has remarkable potential for various applications (e.g., daily-living activity monitoring, motion analysis for sports enhancement). However, no existing work has tackled this task due to the difficulty of estimating a human pose from a single camera image in which only a part of the human body is captured and the lack of training data.
- Categories:
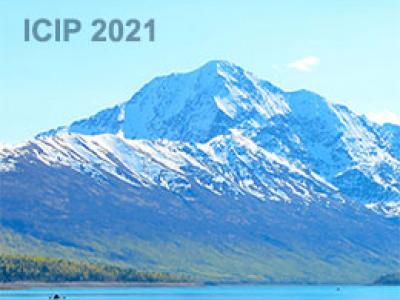
- Read more about An Efficient Image Compression Method Based On Neural Network: An Overfitting Approach
- Log in to post comments
Over the past decade, nonlinear image compression techniques based on neural networks have been rapidly developed to achieve more efficient storage and transmission of images compared with conventional linear techniques. A typical non-linear technique is implemented as a neural network trained on a vast set of images, and the latent representation of a target image is transmitted. In contrast to the previous nonlinear techniques, we propose a new image compression method in which a neural network model is trained exclusively on a single target image, rather than a set of images.
slides.pdf

- Categories:
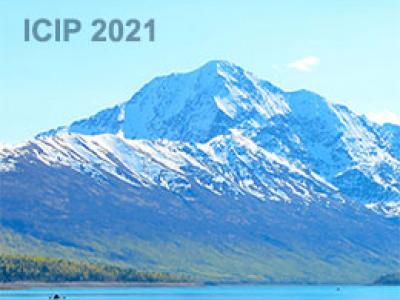
- Read more about Learning to Correct Axial Motion in OCT For 3D Retinal Imaging
- Log in to post comments
Optical Coherence Tomography (OCT) is a powerful technique for non-invasive 3D imaging of biological tissues at high resolution that has revolutionized retinal imaging. A major challenge in OCT imaging is the motion artifacts introduced by involuntary eye movements. In this paper, we propose a convolutional neural network that learns to correct axial motion in OCT based on a single volumetric scan. The proposed method is able to correct large motion, while preserving the overall curvature of the retina.
- Categories:
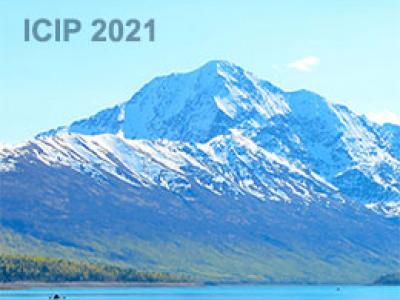
- Read more about Improving filling level classification with adversarial training
- Log in to post comments
- Categories: