Documents
Demo
Joint Global-Local alignment for domain adaptive semantic segmentation
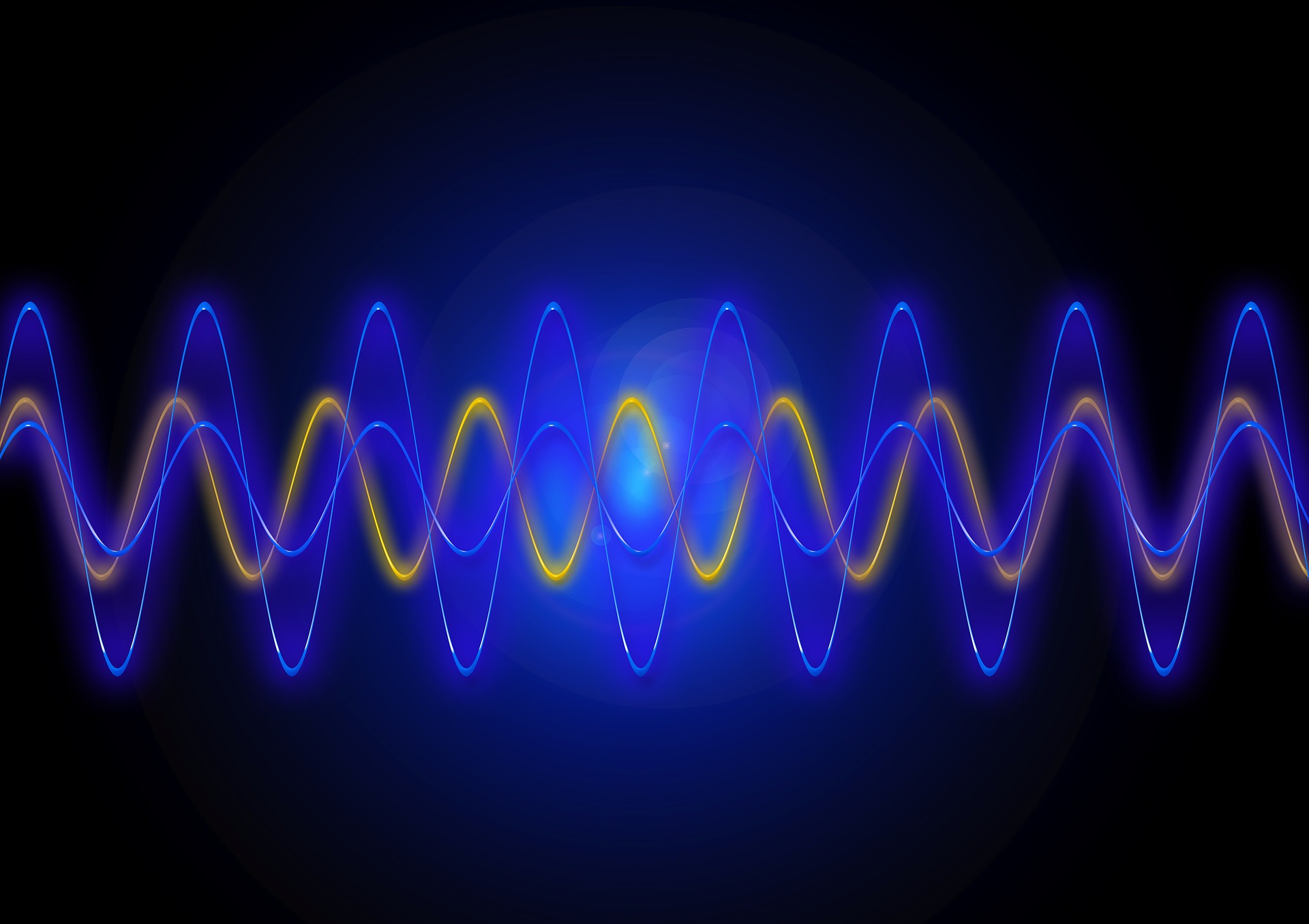
- Citation Author(s):
- Submitted by:
- Sudhir Yarram
- Last updated:
- 6 May 2022 - 2:52am
- Document Type:
- Demo
- Presenters:
- Sudhir Yarram
- Paper Code:
- MLSP-23.5
- Categories:
- Log in to post comments
Unsupervised domain adaptation has shown promising results in leveraging synthetic (source) images for semantic segmentation of real (target) images. One key issue is how to align data distributions between the source and target domains. Adversarial learning has been applied to align these distributions. However, most existing approaches focus on aligning the output distributions related to image (global) segmentation. Such global alignment may not result in effective alignment due to the inherent high dimensionality feature space involved in the alignment. Moreover, global alignment might be hindered by the noisy outputs corresponding to background pixels in the source domain. To address this limitation, we propose a local output alignment. Such an approach can also mitigate the influences of noisy background pixels from the source domain when performing the local alignment. Our experiments show that by adding local output alignment into various global alignment based domain adaptation, our joint global-local alignment methods improves semantic segmentation. Code is available at https://github.com/skrya/globallocal.