Documents
Presentation Slides
JOINTLY LEARNING SELECTION MATRICES FOR TRANSMITTERS, RECEIVERS AND FOURIER COEFFICIENTS IN MULTICHANNEL IMAGING
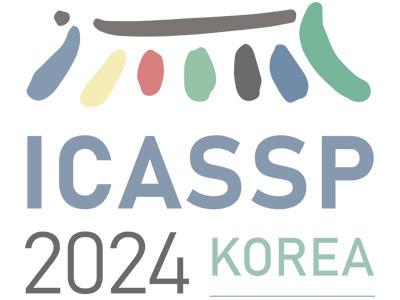
- Citation Author(s):
- Submitted by:
- Han Wang
- Last updated:
- 15 April 2024 - 1:07am
- Document Type:
- Presentation Slides
- Document Year:
- 2024
- Event:
- Presenters:
- Han Wang
- Paper Code:
- SAM-L3.4
- Categories:
- Log in to post comments
Strategic subsampling has become a focal point due to its effectiveness in compressing data, particularly in the Full Matrix Capture (FMC) approach in ultrasonic imaging. This paper introduces the Joint Deep Probabilistic Subsampling (J-DPS) method, which aims to learn optimal selection matrices simultaneously for transmitters, receivers, and Fourier coefficients. This task-based algorithm is realized by introducing a specialized measurement model and integrating a customized Complex Learned FISTA (CL-FISTA) network. We propose a parallel network architecture, partitioned into three segments corresponding to the three matrices, all working toward a shared optimization objective with adjustable loss allocation. A synthetic dataset is designed to reflect practical scenarios, and we provide quantitative comparisons with a traditional CRB-based algorithm, standard DPS, and J-DPS.