Documents
Poster
Knowledge Distillation Using Output Errors for Self-Attention ASR Models
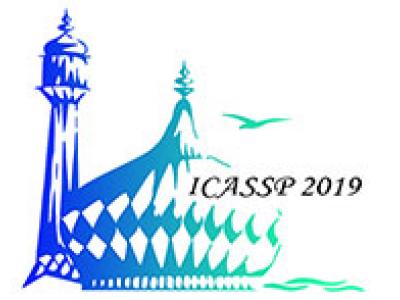
- Citation Author(s):
- Submitted by:
- Ho-Gyeong Kim
- Last updated:
- 8 May 2019 - 10:02pm
- Document Type:
- Poster
- Document Year:
- 2019
- Event:
- Presenters:
- Hwidong Na
- Categories:
- Log in to post comments
Most automatic speech recognition (ASR) neural network models are not suitable for mobile devices due to their large model sizes. Therefore, it is required to reduce the model size to meet the limited hardware resources. In this study, we investigate sequence-level knowledge distillation techniques of self-attention ASR models for model compression. In order to overcome the performance degradation of compressed models, our proposed method adds an exponential weight to the sequence-level knowledge distillation loss function, which reflects the word error rate of the output of the teacher model based on the ground-truth word sequences. Evaluated on LibriSpeech dataset, the proposed knowledge distillation method achieves significant improvements over the student baseline model.