Documents
Poster
KPSNET: KEYPOINT DETECTION AND FEATURE EXTRACTION FOR POINT CLOUD REGISTRATION
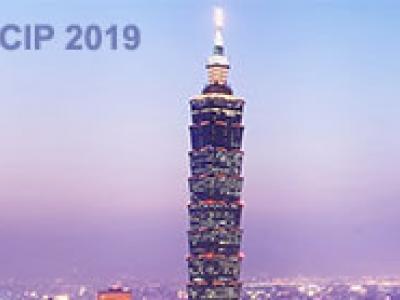
- Citation Author(s):
- Submitted by:
- Anan Du
- Last updated:
- 16 September 2019 - 9:58pm
- Document Type:
- Poster
- Document Year:
- 2019
- Event:
- Presenters:
- Anan DU
- Paper Code:
- 3546
- Categories:
- Log in to post comments
This paper presents the KPSNet, a KeyPoint Siamese Network to simultaneously learn task-desirable keypoint detector and feature extractor. The keypoint detector is optimized to predict a score vector, which signifies the probability of each candidate being a keypoint. The feature extractor is optimized to learn robust features of keypoints by exploiting the correspondence between the keypoints generated from two inputs, respectively. For training, the KPSNet does not require to manually annotate keypoints and local patches pairwise. Instead, we design an alignment module to establish the correspondence between the two inputs and generate positive and negative samples on-the-fly. Therefore, our method can be easily extended to new scenes. We test the proposed method on the open-source benchmark and experiments show the validity of our method.