Documents
Poster
Langevin-based Strategy for Efficient Proposal Adaptation in Population Monte Carlo
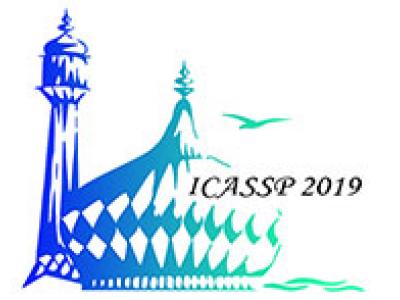
- Citation Author(s):
- Submitted by:
- Emilie Chouzenoux
- Last updated:
- 8 May 2019 - 8:22am
- Document Type:
- Poster
- Document Year:
- 2019
- Event:
- Presenters:
- Emilie Chouzenoux
- Paper Code:
- SPTM-P2.10
- Categories:
- Log in to post comments
Population Monte Carlo (PMC) algorithms are a family of
adaptive importance sampling (AIS) methods for approximating
integrals in Bayesian inference. In this paper, we propose
a novel PMC algorithm that combines recent advances
in the AIS and the optimization literatures. In such a way, the
proposal densities are adapted according to the past weighted
samples via a local resampling that preserves the diversity,
but we also exploit the geometry of the targeted distribution.
A scaled Langevin strategy with Newton-based scaling metric
is retained for this purpose, allowing to adapt jointly the
means and the covariances of the proposals, without needing
to tune any extra parameter. The performance of the proposed
technique is clearly superior in two numerical examples at the
cost of a reasonable computational complexity increment.