Documents
Presentation Slides
LANGUAGE-FREE COMPOSITIONAL ACTION GENERATION VIA DECOUPLING REFINEMENT
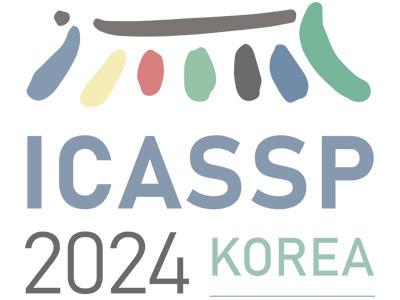
- DOI:
- 10.60864/fhd5-es04
- Citation Author(s):
- Submitted by:
- Xiao Liu
- Last updated:
- 6 June 2024 - 10:28am
- Document Type:
- Presentation Slides
- Event:
- Categories:
- Log in to post comments
Composing simple actions into complex actions is crucial yet challenging. Existing methods largely rely on language annotations to discern composable latent semantics, which is costly and labor-intensive. In this study, we introduce a novel framework to generate compositional actions without language auxiliaries. Our approach consists of three components: Action Coupling, Conditional Action Generation, and Decoupling Refinement. Action Coupling integrates two subactions to generate pseudo-training examples. Then, a conditional generative model, CVAE is employed to facilitate the diverse generation. Decoupling Refinement leverages a self-supervised pre-trained model MAE to ensure semantic consistency between sub-actions and compositional actions. Due to the lack of existing datasets containing both sub-actions and compositional actions, we create two new datasets, named HumanAct-C and UESTC-C. Both qualitative and quantitative assessments are conducted to show our efficacy.
Comments
Presentation slides for
Presentation slides for ICASSP 2024