Documents
Presentation Slides
LEARNED IMAGE COMPRESSION GUIDED ADAPTIVE QUANTIZATION FOR PERCEPTUAL QUALITY
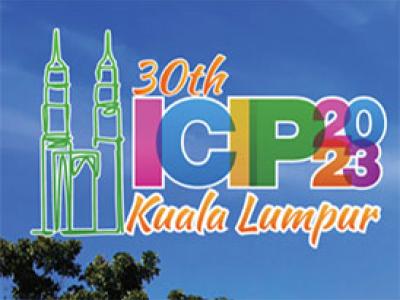
- DOI:
- 10.60864/y2bp-1d47
- Citation Author(s):
- Submitted by:
- Cheng Chen
- Last updated:
- 17 November 2023 - 12:05pm
- Document Type:
- Presentation Slides
- Document Year:
- 2023
- Event:
- Presenters:
- Cheng Chen
- Categories:
- Log in to post comments
Neural network based image compression has made significant progress in recent years. The learned image codecs are commonly reported to outperform their conventional counterparts in perceptual quality. Despite the superior performance, the learned image codecs are much more complex to decode, which hinders their usage in practice. Without a significant advance in hardware capability, the conventional image codec will likely remain a primary component for large scale image services. It is therefore desirable to improve the quality of conventional image codecs. In this paper, we present an adaptive quantization approach to the conventional image codec with the help of learned image codecs to improve its perceptual quality. It exploits the bit allocation of the neural network based image codec to adapt the quantizers on a block basis. It is experimentally shown that the proposed method provides considerable perceptual quality improvements over other leading contenders.