Documents
Poster
Poster
LEARNING GEOGRAPHICALLY DISTRIBUTED DATA FOR MULTIPLE TASKS USING GENERATIVE ADVERSARIAL NETWORKS
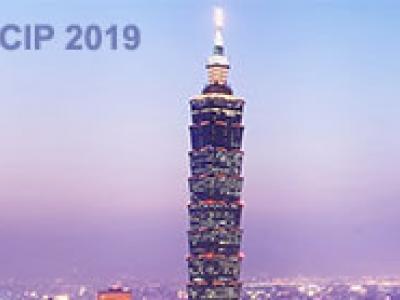
- Citation Author(s):
- Submitted by:
- Xiaoqing Zhu
- Last updated:
- 16 September 2019 - 11:19am
- Document Type:
- Poster
- Document Year:
- 2019
- Event:
- Presenters:
- Yaqi Wang, Xiaoqing Zhu, and Wai-tian Tan
- Paper Code:
- 2489
- Categories:
- Keywords:
- Log in to post comments
We present a novel method that supports the learning of multiple classification tasks from geographically distributed data. By combining locally trained generative adversarial networks (GANs) with a small fraction of original data samples, our proposed scheme can train multiple discriminative models at a central location with low communication overhead. Experiments using common image datasets (MNIST, CIFAR-10, LSUN-20, Celeb-A) show that our proposed scheme can achieve comparable classification accuracy as the ideal classifier trained using all data from all sites. We further demonstrate that our method can scale to 10 sites without sacrificing classification accuracy for large datasets such as LSUN-20.
poster-v1.pdf
