Documents
Supplemental Material
Learning From PU Data Using Disentangled Representations [Supplemental Material]
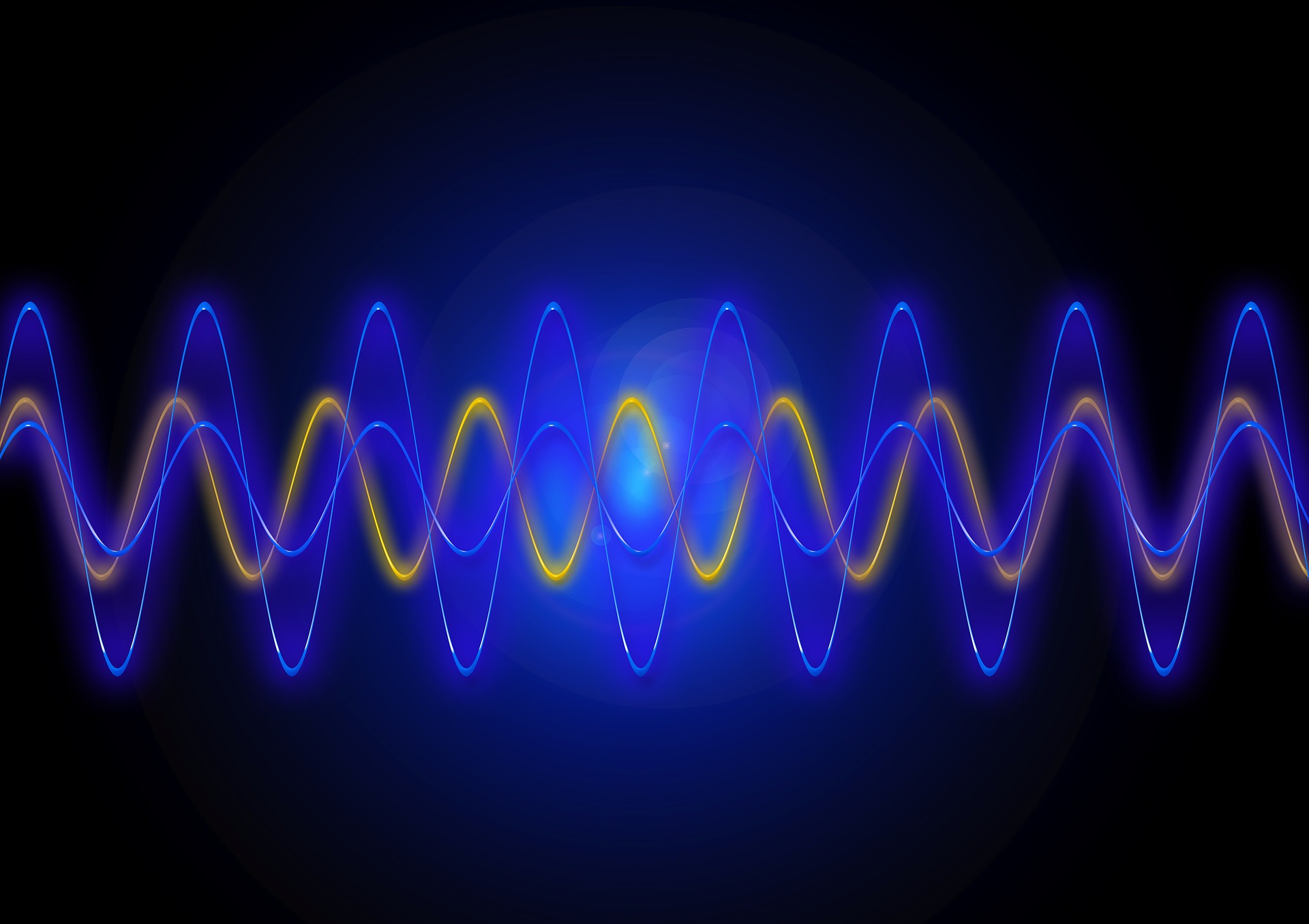
- Citation Author(s):
- Submitted by:
- Anonymous Submission
- Last updated:
- 4 February 2025 - 3:24pm
- Document Type:
- Supplemental Material
- Categories:
- Log in to post comments
We address the problem of learning a binary classifier given partially labeled data where all labeled samples come from only one of the classes, commonly known as Positive Unlabeled (PU) learning. Classical methods such as clustering, out-of-distribution detection, and positive density estimation, while effective in low-dimensional scenarios, lose their efficacy as the dimensionality of data increases, because of the increasing complexity. The contribution of this paper is the learning of a neural network-based data representation by employing a loss function that enables the projection of unlabeled data into two distinct clusters - positive and negative - facilitating their identification through basic clustering techniques and mirroring the simplicity of the problem seen in low-dimensional settings. Our experimental results on benchmarking PU datasets validate the superiority of our method over existing state-of-the-art techniques. Additionally, we provide theoretical justification to support our cluster-based approach and algorithmic choices.