Documents
Presentation Slides
Presentation Slides
LEARNING SIGNED GRAPHS FROM DATA
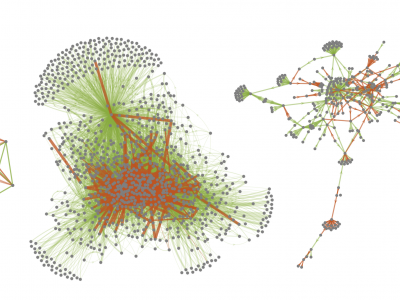
- Citation Author(s):
- Submitted by:
- Gerald Matz
- Last updated:
- 14 May 2020 - 5:04am
- Document Type:
- Presentation Slides
- Document Year:
- 2020
- Event:
- Presenters:
- Gerald Matz
- Paper Code:
- SPTM-P1.10
- Categories:
- Log in to post comments
Signed graphs have recently been found to offer advantages over unsigned graphs in a variety of tasks. However, the problem of learning graph topologies has only been considered for the unsigned case. In this paper, we propose a conceptually simple and flexible approach to signed graph learning via signed smoothness metrics. Learning the graph amounts to solving a convex optimization problem, which we show can be reduced to an efficiently solvable quadratic problem. Applications to signal reconstruction and clustering corroborate the effectiveness of the proposed method.