Documents
Presentation Slides
LEARNING SPATIALLY-CORRELATED TEMPORAL DICTIONARIES FOR CALCIUM IMAGING
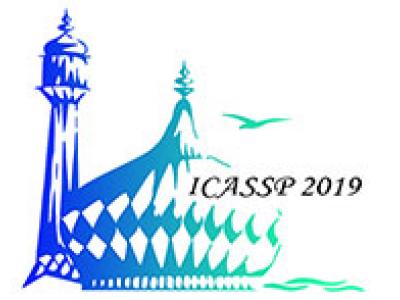
- Citation Author(s):
- Submitted by:
- Gal Mishne
- Last updated:
- 9 May 2019 - 4:34pm
- Document Type:
- Presentation Slides
- Document Year:
- 2019
- Event:
- Presenters:
- Gal Mishne
- Paper Code:
- 2519
- Categories:
- Log in to post comments
Calcium imaging has become a fundamental neural imaging technique, aiming to recover the individual activity of hundreds of neurons in a cortical region. Current methods (mostly matrix factorization) are aimed at detecting neurons in the field-of-view and then inferring the corresponding time-traces. In this paper, we reverse the modeling and instead aim to minimize the spatial inference, while focusing on finding the set of temporal traces present in the data. We reframe the problem in a dictionary learning setting, where the dictionary contains the time-traces and the sparse coefficient are spatial maps. We adapt dictionary learning to calcium imaging by introducing constraints on the norms and correlations of the time-traces, and incorporating a hierarchical spatial filtering model that correlates the time-trace usage over the field-of-view. We demonstrate
on synthetic and real data that our solution has advantages regarding initialization, implicitly inferring number of neurons and simultaneously detecting different neuronal types.
ICASSP.pdf
