Documents
Poster
LEARNING SPATIO-TEMPORAL RELATIONS WITH MULTI-SCALE INTEGRATED PERCEPTION FOR VIDEO ANOMALY DETECTION
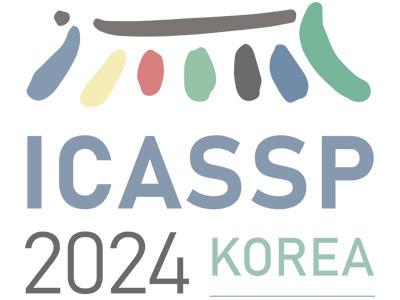
- DOI:
- 10.60864/egag-5563
- Citation Author(s):
- Submitted by:
- Hongyu Ye
- Last updated:
- 6 June 2024 - 10:28am
- Document Type:
- Poster
- Document Year:
- 2024
- Event:
- Presenters:
- Hongyu Ye
- Paper Code:
- IVMSP-P1.11
- Categories:
- Log in to post comments
In weakly supervised video anomaly detection, it has been verified that anomalies can be biased by background noise. Previous works attempted to focus on local regions to exclude irrelevant information. However, the abnormal events in different scenes vary in size, and current methods struggle to consider local events of different scales concurrently. To this end, we propose a multi-scale integrated perception
(MSIP) learning approach to perceive abnormal regions of different scales simultaneously. In our method, a frame is partitioned into several groups of patches with varying scales, and a multi-scale patch spatial relation (MPSR) module is further proposed to model the inconsistencies among multi-scale patches. Specifically, we design a hierarchical graph convolution block in the MPSR module to improve the integration
of patch features by implementing cross-scale feature learning. An existing clip temporal relation network is also introduced to enable spatio-temporal encoding in our model. Experiments show that our method achieves new state-of-the-art performance on the ShanghaiTech and competitive results on UCF-Crime benchmarks.