Documents
Poster
Less is More: Compression of Deep Neural Networks for adaptation in photonic FPGA circuits
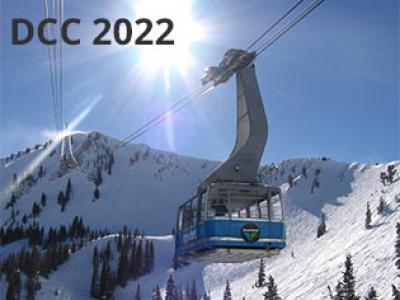
- Citation Author(s):
- Submitted by:
- Eftychia Makri
- Last updated:
- 8 March 2022 - 4:24am
- Document Type:
- Poster
- Event:
- Presenters:
- Eftychia Makri
- Categories:
- Log in to post comments
Photonic circuits pave the way to ultrafast computing and real-time inference of applications with paramount importance, such as imaging flow cytometry (IFC). However, current implementations exhibit inherent restrictions that consequently diminish the neural networks (NN) complexity that can be supported. Thus, NN compression mechanisms are deemed critical for the efficient deployment of such demanding tasks. In this work, several compression schemes are thoroughly investigated under the prism of photonic field-programmable gate array (FPGA) limitations to reveal the most appropriate candidate to address real end-users needs maintaining enhanced level of detection accuracy. Among them, the combined approach of convolutional auto-encoder, pruning, quantization and knowledge distillation exhibits the best performance revealing its potential for accelerated on-site inference.