Documents
Poster
Leveraging LSTM Models for Overlap Detection in Multi-Party Meetings
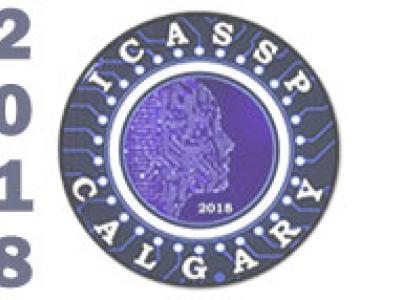
- Citation Author(s):
- Submitted by:
- Neeraj Sharma
- Last updated:
- 14 April 2018 - 2:54am
- Document Type:
- Poster
- Document Year:
- 2018
- Event:
- Presenters:
- SRIRAM GANAPATHY
- Paper Code:
- 4021
- Categories:
- Log in to post comments
The detection of overlapping speech segments is of key importance in speech applications involving analysis of multi-party conversations. The detection problem is challenging because overlapping speech segments are typically captured as short speech utterances far-field microphone recordings. In this paper, we propose detection of overlap segments using a neural network architecture consisting of long-short term memory (LSTM) models. The neural network architecture learns the presence of overlap in speech by identifying the spectrotemporal structure of overlapping speech segments. In order to evaluate the model performance, we perform experiments on simulated overlapped speech generated from the TIMIT database, and natural multi-talker conversational speech in the augmented multi-party interaction (AMI) meeting corpus. The proposed approach yields improvements over a Gaussian mixture model based overlap detection system. Furthermore, as an application of overlap detection, integration of overlap detection into speaker diarization task is shown to give improvement in diarization error rate.