Documents
Poster
A LIGHTWEIGHT NEURAL NETWORK FOR CROWD ANALYSIS OF IMAGES WITH CONGESTED SCENES
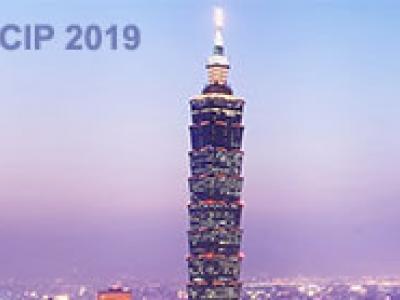
- Citation Author(s):
- Submitted by:
- Shan Du
- Last updated:
- 11 September 2019 - 2:29pm
- Document Type:
- Poster
- Document Year:
- 2019
- Event:
- Presenters:
- Shan Du
- Paper Code:
- 2274
- Categories:
- Log in to post comments
For images with congested scenes, the task of crowd analysis,
including crowd counting and crowd distribution prediction,
becomes very difficult. To address these issues, various
CNN-based approaches have been proposed. However, those
methods usually have a large number of parameters and require
huge computing resources. In this paper, we focus on
low-complexity approaches and propose a lightweight endto-
end network for crowd analysis. Our method utilizes an
effective scale-aware module to extract multi-scale features
and then regresses these features to density maps. The proposed
network is consisted by three parts: multi-scale feature
extraction, density map estimation and density map correction,
and the network which only contains 0.86 M parameters
(Lightweight). According to our experiments, our proposal
obtains a better result than other existing methods on several
testing sequences.