Documents
Presentation Slides
Location-Relative Attention Mechanisms For Robust Long-Form Speech Synthesis
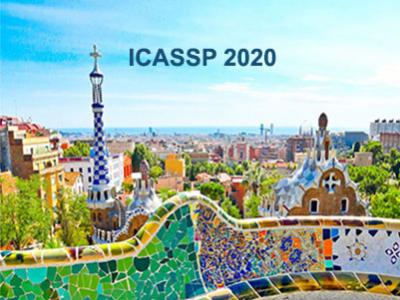
- Citation Author(s):
- Submitted by:
- Eric Battenberg
- Last updated:
- 14 May 2020 - 6:30pm
- Document Type:
- Presentation Slides
- Document Year:
- 2020
- Event:
- Presenters:
- Eric Battenberg
- Paper Code:
- SPE-L5.4
- Categories:
- Log in to post comments
Despite the ability to produce human-level speech for in-domain text, attention-based end-to-end text-to-speech (TTS) systems suffer from text alignment failures that increase in frequency for out-of-domain text. We show that these failures can be addressed using simple location-relative attention mechanisms that do away with content-based query/key comparisons. We compare two families of attention mechanisms: location-relative GMM-based mechanisms and additive energy-based mechanisms. We suggest simple modifications to GMM-based attention that allow it to align quickly and consistently during training, and introduce a new location-relative attention mechanism to the additive energy-based family, called Dynamic Convolution Attention (DCA). We compare the various mechanisms in terms of alignment speed and consistency during training, naturalness, and ability to generalize to long utterances, and conclude that GMM attention and DCA can generalize to very long utterances, while preserving naturalness for shorter, in-domain utterances.