Documents
Presentation Slides
Low-Complexity Compressed Analysis in Eigenspace with Limited Labeled Data for Real-Time Electrocardiography Telemonitoring
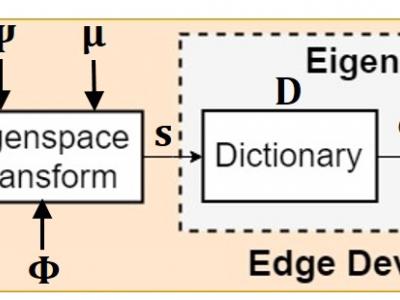
- Citation Author(s):
- Submitted by:
- Kai-Chieh Hsu
- Last updated:
- 12 December 2018 - 10:09pm
- Document Type:
- Presentation Slides
- Document Year:
- 2018
- Event:
- Presenters:
- Kai-Chieh Hsu
- Paper Code:
- BIO-L.2.4
- Categories:
- Log in to post comments
To achieve real-time electrocardiography (ECG) telemonitoring, we need to overcome the scarce bandwidth. Compressed sensing (CS) emerges as a promising technique to greatly compress ECG signal with little computation. Furthermore, with edge-classification, we can reduce the data rate by transmitting abnormal ECG signals only. However, there are three main limitations: limited number of labeled ECG signal, tight battery constraint of edge devices and low response time requirement. Task-driven dictionary learning (TDDL) appears as an appropriate classifier to render low complexity and high generalization. Combining CS with TDDL directly (CA-N) will degrade classification and need higher complexity model. In this paper, we proposed an eigenspace-aided compressed analysis (CA-E) integrating principal component analysis (PCA), CS and TDDL, sustaining not only light complexity but high performance under exiguous labeled ECG data set. Simulation results show CA-E reduces about 67% parameters, 76% training time, 87% inference time and has the smaller accuracy variance to CA-N counterpart.