Documents
Poster
Low Dose Abdominal CT Image Reconstruction: An Unsupervised Learning based Approach
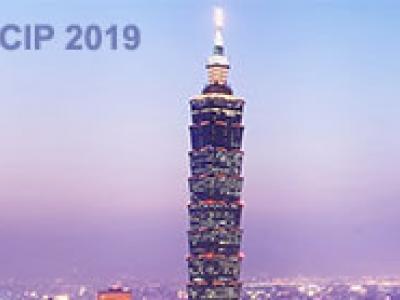
- Citation Author(s):
- Submitted by:
- Shiba Kuanar
- Last updated:
- 24 September 2019 - 10:23am
- Document Type:
- Poster
- Document Year:
- 2019
- Event:
- Presenters:
- Shiba Kuanar
- Paper Code:
- 1720
- Categories:
- Log in to post comments
In medical practice, the X-ray Computed tomography-based scans expose a high radiation dose and lead to the risk of prostate or abdomen cancers. On the other hand, the low-dose CT scan can reduce radiation exposure to the patient. But the reduced radiation dose degrades image quality for human perception, and adversely affects the radiologist’s diagnosis and prognosis. In this paper, we introduce a GAN based auto-encoder network to de-noise the CT images. Our network first maps CT images to low dimensional manifolds and then restore the images from its corresponding manifold representations. Our reconstruction algorithm separately calculates perceptual similarity, learns the latent feature maps, and achieves more accurate and visually pleasing reconstructions. We also showed the effectiveness of our model on a number of patient abdomen CT images, and compare our results with existing deep learning and iterative reconstruction methods. Experimental results demonstrate that our model outperforms other state-of-the-art methods in terms of PSNR, SSIM, and statistical properties of the image regions.