Documents
Presentation Slides
M3VSNet: Unsupervised Multi-metric Multi-view Stereo Network
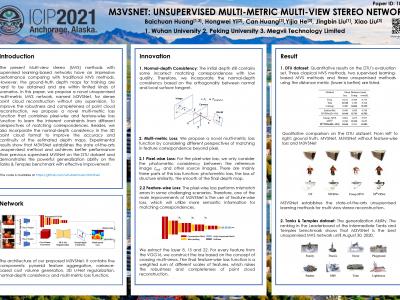
- Citation Author(s):
- Submitted by:
- Baichuan Huang
- Last updated:
- 23 September 2021 - 8:23pm
- Document Type:
- Presentation Slides
- Document Year:
- 2021
- Event:
- Presenters:
- Baichuan Huang
- Paper Code:
- 1091
- Categories:
- Log in to post comments
The present Multi-view stereo (MVS) methods with supervised learning-based networks have an impressive performance comparing with traditional MVS methods. However, the ground-truth depth maps for training are hard to be obtained and are within limited kinds of scenarios. In this paper, we propose a novel unsupervised multi-metric MVS network, named M^3VSNet, for dense point cloud reconstruction without any supervision. To improve the robustness and completeness of point cloud reconstruction, we propose a novel multi-metric loss function that combines pixel-wise and feature-wise loss function to learn the inherent constraints from different perspectives of matching correspondences. Besides, we also incorporate the normal-depth consistency in the 3D point cloud format to improve the accuracy and continuity of the estimated depth maps. Experimental results show that M3VSNet establishes the state-of-the-arts unsupervised method and achieves comparable performance with previous supervised MVSNet on the DTU dataset and demonstrates the powerful generalization ability on the Tanks and Temples benchmark with effective improvement. Our code is available at https://github.com/whubaichuan/M3VSNet .
slide1091.pdf
