Documents
Presentation Slides
MAP-informed Unrolled Algorithms for Hyper-parameter Estimation
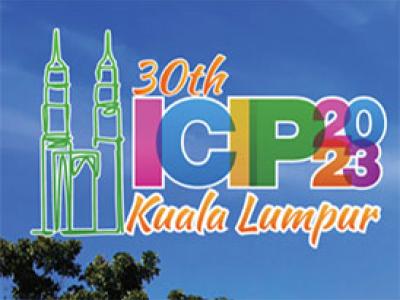
- DOI:
- 10.60864/sfgc-b752
- Citation Author(s):
- Submitted by:
- Caroline Chaux
- Last updated:
- 17 November 2023 - 12:05pm
- Document Type:
- Presentation Slides
- Document Year:
- 2023
- Event:
- Presenters:
- Caroline Chaux
- Categories:
- Log in to post comments
Hyper-parameter tuning, and especially regularisation parameter estimation, is a challenging but essential task when solving inverse problems. The solution is obtained here through the minimization of a functional composed of a data fidelity term and a regularization term. Those terms are balanced through a (or several) regularisation parameter(s) whose estimation is made under an unrolled strategy together with the inverse problem solving. The resulting network is
trained while incorporating information on the model through Maximum a Posteriori estimation which drastically decreases the amount of data needed for the training and results in better estimation results. The performances are demonstrated in a deconvolution context where the regularisation is performed in the wavelet domain.
ICIP_oral.pdf
