Documents
Poster
MARGIN-EMBEDDING CANONICAL CORRELATION ANALYSIS WITH FEATURE SELECTION FOR PERSON RE-IDENTIFICATION
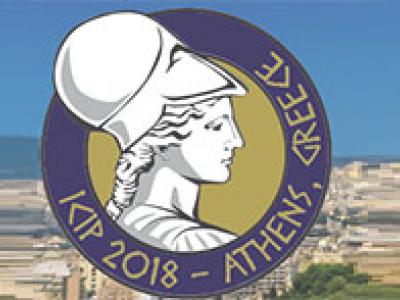
- Citation Author(s):
- Submitted by:
- Linfei Ma
- Last updated:
- 5 October 2018 - 1:09pm
- Document Type:
- Poster
- Document Year:
- 2018
- Event:
- Presenters:
- Linfei Ma
- Paper Code:
- 1749
- Categories:
- Log in to post comments
Canonical correlation analysis (CCA) is a classical subspace learning method of capturing the common semantic information underlying multi-view data. It has been used in person re-identification (re-ID) task by treating the task of matching identical individuals across non-overlapping multi-cameras as a multi-view learning problem. However, CCA-based re-ID methods still achieve unsatisfactory results because few jointly consider discriminative margin information and selecting importantly relevant features. To address this issue, we propose a novel l2,1-norm regularized margin-embedding CCA (l2,1-MCCA), which learns a generalized discriminative subspace by employing more discriminative margin information. Moreover, the new method enforces the l2,1-norm regularization term over the learned subspace to identify the relevant features. Both lightweight and effective schemes can benefit from each other and endeavor to enlarge the interclass variations whilst reducing the intra-class variations. Experiments on three popular datasets show the efficacy of l2,1-MCCA as compared with recently representative re-ID methods.