Documents
Presentation Slides
Matched Manifold Detection for Group-Invariant Registration and Classification of Images
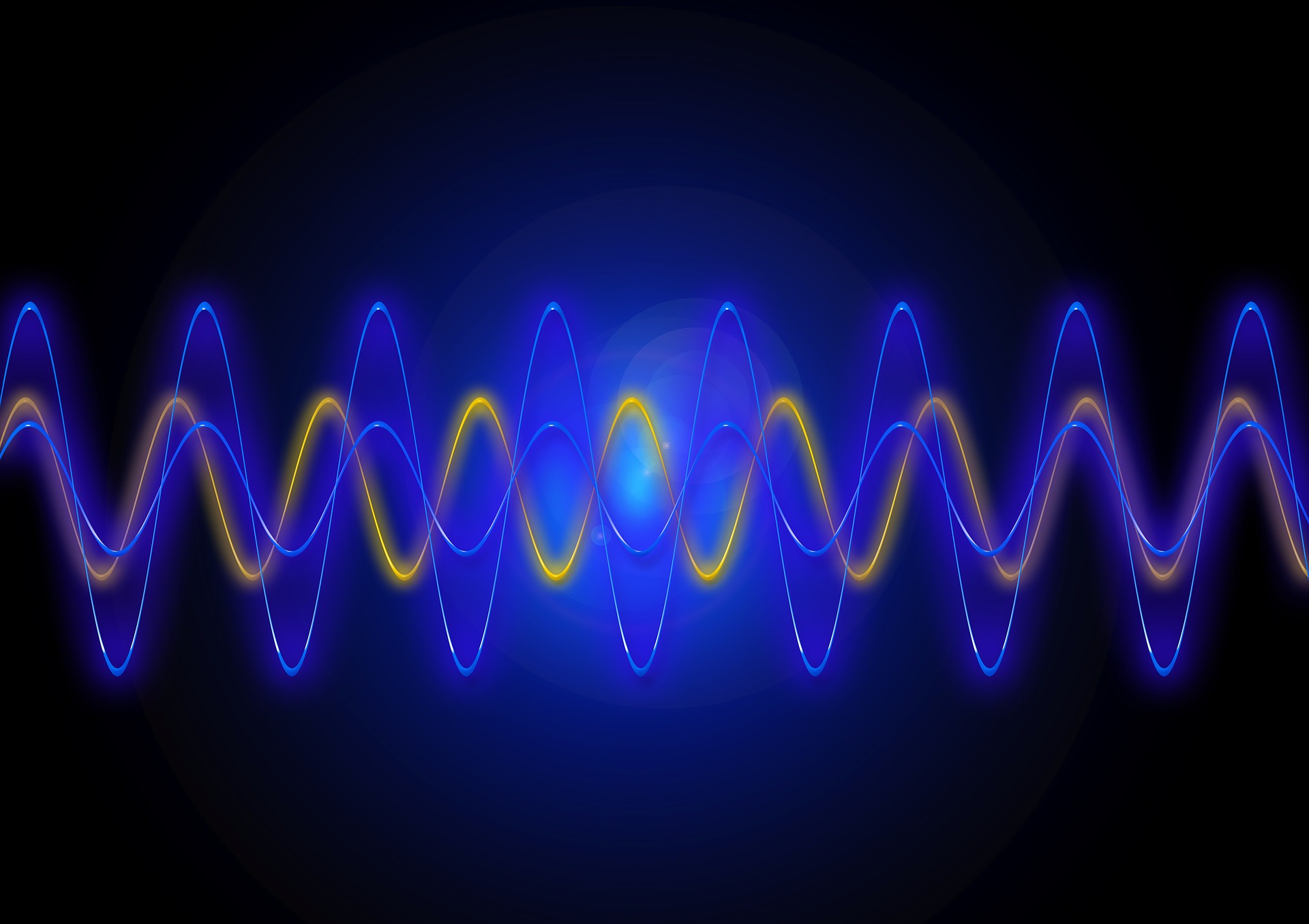
- Citation Author(s):
- Submitted by:
- Joseph Francos
- Last updated:
- 15 May 2022 - 7:05am
- Document Type:
- Presentation Slides
- Paper Code:
- SS-14.5
- Categories:
- Log in to post comments
Consider the set of possible observations turned out by geometric and radiometric transformations of an object. This set is generally a manifold in the ambient space of observations. It has been shown [1] that in those cases where the geometric deformations are affine and the radiometric deformations are monotonic, the radiometry invariant universal manifold embedding (RIUME) provides a mapping from the orbit of deformed observations to a single low dimensional linear subspace of Euclidean space. This linear subspace is invariant to the geometric and radiometric transformations and hence is a representative of the orbit. It thus naturally serves as an invariant statistic for solving problems of joint transformation estimation and detection or classification. In the unsupervised detection problem, subspaces evaluated from two observations are tested for the similarity of the observed object and their relative transformation is estimated from the RIUME matrix representation. In the classification set-up the RIUME subspace extracted from an experimental observation is tested against a set of subspaces representing the different object manifolds, in search for the nearest class. We show how to extract a set of mutually orthogonal subspaces, where each subspace represents a different object manifold. In the presence of observation noise, the observations do not lie strictly on the manifold and the resulting RIUME subspaces are noisy. We derive a method for estimating the mean subspace representation of a manifold of deformed observations. To optimize the performance of the matched manifold detector in the presence of observation noise, an analytic solution for choosing the RIUME nonlinear operators is derived, achieving the effect of simultaneous denoising of the object manifolds. The invariant representation of the object is the basis of a matched manifold detection and tracking framework for objects that undergo complex geometric and radiometric deformations. The experimental results on natural scenes demonstrate the generality and applicability of the RIUME framework for classification, detection, and dense registration.