Documents
Presentation Slides
MAXIMUM LIKELIHOOD-BASED GRIDLESS DOA ESTIMATION USING STRUCTURED COVARIANCE MATRIX RECOVERY AND SBL WITH GRID REFINEMENT
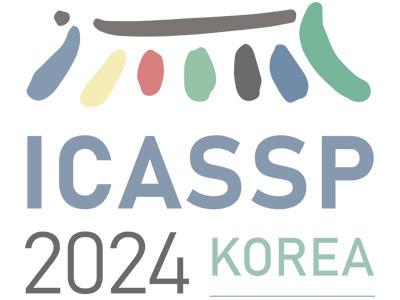
- DOI:
- 10.60864/jm0x-qm58
- Citation Author(s):
- Submitted by:
- Rohan Ramchandr...
- Last updated:
- 15 April 2024 - 2:41am
- Document Type:
- Presentation Slides
- Document Year:
- 2024
- Event:
- Presenters:
- Bhaskar D. Rao
- Paper Code:
- 11551
- Categories:
- Log in to post comments
We consider the parametric measurement model employed in applications such as line spectral or direction-of-arrival estimation with the goal to estimate the underlying parameter in a gridless manner. We focus on the stochastic maximum likelihood estimation (MLE) framework and overcome the model complexities of the past by reparameterization of the objective and exploiting the sparse Bayesian learning (SBL) approach. SBL is shown to be a correlation-aware method and, for the underlying problem, a grid-based technique for recovering a structured covariance matrix of the measurements. For the case when measurements are spatial (or temporal) samples at regular intervals, the structured matrix is expressible as a sampled Toeplitz matrix. In this case, additional constraints and reparameterization of the SBL objective leads to the proposed structured matrix recovery technique based on MLE. The optimization problem is non-convex and a majorization-minimization based iterative procedure is proposed to estimate the structured matrix; each iteration solves a semidefinite program. We recover the parameter of interest in a gridless manner by appealing to the Carathéodory-Féjer result on decomposition of positive semidefinite Toeplitz matrices. For the general case of irregularly spaced samples, we propose an iterative SBL procedure that refines grid points to increase resolution near potential source locations, while maintaining a low per iteration complexity. We provide numerical results to compare the performance of the proposed techniques with other gridless techniques, and the Cramér-Rao bound. The proposed correlation-aware approach is more robust to issues such as fewer snapshots, correlated or closely separated sources, and improves sources identifiability.