Documents
Presentation Slides
Meta Learning for Robust Child/Adult Classification from Speech
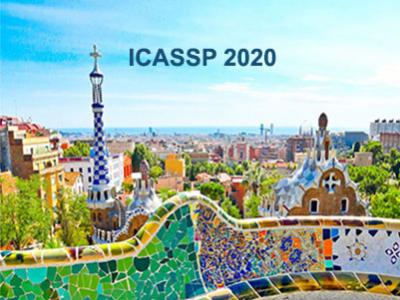
- Citation Author(s):
- Submitted by:
- Manoj Kumar
- Last updated:
- 13 May 2020 - 5:07pm
- Document Type:
- Presentation Slides
- Document Year:
- 2020
- Event:
- Presenters:
- Manoj Kumar
- Paper Code:
- ICASSP 3791
- Categories:
- Log in to post comments
Computational modeling of naturalistic conversations in clinical applications has seen growing interest in the past decade. An important use-case involves child-adult interactions within the autism diagnosis and intervention domain. In this paper, we address a specific sub-problem of speaker diarization, namely child-adult speaker classification in such dyadic conversations with specified roles. Training a speaker classification system robust to speaker and channel conditions is challenging due to inherent variability in the speech within children and the adult interlocutors. In this work, we propose the use of meta-learning, in particular prototypical networks which optimize a metric space across multiple tasks. By modeling every child-adult pair in the training set as a separate task during meta-training, we learn a representation with improved generalizability compared to conventional supervised learning. We demonstrate improvements over state-of-the-art speaker embeddings (x-vectors) under two evaluation settings: weakly supervised classification (upto 14.53% relative improvement in F1-scores) and clustering (upto relative 9.66% improvement in cluster purity). Our results show that protonets can potentially extract robust speaker embeddings for child-adult classification from speech.