Documents
Poster
Motivating bilevel approaches to filter learning: A case study
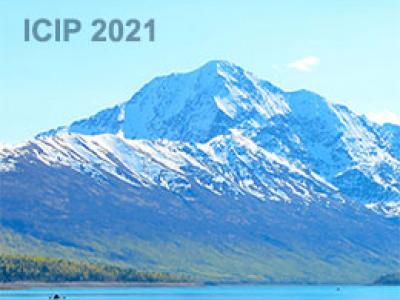
- Citation Author(s):
- Submitted by:
- Caroline Crockett
- Last updated:
- 27 September 2021 - 5:13am
- Document Type:
- Poster
- Document Year:
- 2021
- Event:
- Presenters:
- Caroline Crockett
- Categories:
- Log in to post comments
The recent trend in regularization methods for inverse problems is to replace handcrafted sparsifying operators with data-driven approaches. Although using such machine learning techniques often improves image reconstruction methods, the results can depend significantly on the learning methodology. This paper compares two supervised learning methods. First, the paper considers a transform learning approach and, to learn the transform, introduces a variant on the Procrustes method for wide matrices with orthogonal rows. Second, we consider a bilevel convolutional filter learning approach. Numerical experiments show the learned transform performs worse for denoising than both the handcrafted finite difference transform and the learned filters, which perform similarly. Our results motivate the use of bilevel learning.