Documents
Poster
MTA: A Lightweight Multilingual Text Alignment Model for Cross-language Visual Word Sense Disambiguation
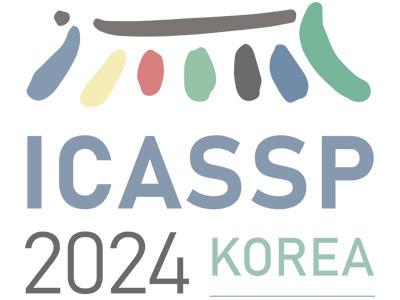
- Citation Author(s):
- Submitted by:
- Qihao Yang
- Last updated:
- 8 April 2024 - 9:13am
- Document Type:
- Poster
- Document Year:
- 2024
- Event:
- Presenters:
- Qihao Yang
- Paper Code:
- 8182
- Categories:
- Keywords:
- Log in to post comments
Visual Word Sense Disambiguation (Visual-WSD), as a subtask of fine-grained image-text retrieval, requires a high level of language-vision understanding to capture and exploit the nuanced relationships between text and visual features. However, the cross-linguistic background only with limited contextual information is considered the most significant challenges for this task. In this paper, we propose MTA, which employs a new approach for multilingual contrastive learning with self-distillation to align fine-grained textual features to fixed vision features and align non-English textual features to English textual momentum features. It is a lightweight and end-to-end model since it does not require updating the visual encoder or translation operations. Furthermore, a trilingual fine-grained image-text dataset is developed and a ChatGPT API module is integrated to enrich the word senses effectively during the testing phase. Extensive experiments show that MTA achieves state-of-the-art results on the benchmark English, Farsi, and Italian datasets in SemEval-2023 Task 1 and exhibits impressive generalization abilities when dealing with variations in text length and language.