Documents
Poster
MULTI-CHANNEL MULTI-LOSS DEEP LEARNING BASED COMPRESSION MODEL FOR COLOR IMAGES
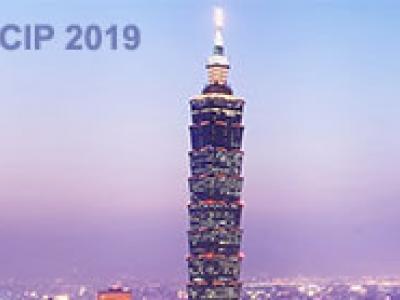
- Citation Author(s):
- Submitted by:
- Phat Nguyen
- Last updated:
- 10 September 2019 - 10:42pm
- Document Type:
- Poster
- Document Year:
- 2019
- Event:
- Presenters:
- Phat
- Paper Code:
- 2902
- Categories:
- Log in to post comments
Lossy image compression aims to encode images with a low bit-rate representation while preserving a pleasant visual quality of decompressed images. By utilizing the manually designed features, the traditional compression may not be suitable for diverse image content and may cause visible artifacts under the low bit rate constraint. Recently, deep learning based methods, which can extract the compact representation of an image in an auto-encoder way, were proposed for image compression. Although satisfying the low bit-rate constraint, they also introduced the color bias problem due to the reconstruction and quantization errors. To overcome these problems, we proposed a deep learning framework to compress an image with two different settings. First, we use separate networks to compress intensity (Y) and color (Cb, Cr) channels. Second, to balance the bit rate and color preservation, we introduce an fusion network, which imports the redundant information from the intensity channel to the color channel. By leveraging the intensity information, the network would focus on disentangling the color-specific features and allow using fewer feature maps to encode the entire image color information. We evaluate the proposed method upon the Kodak image sets by the quantitative metrics (PSNR, SSIM, CM-SSIM). Also, the comparison with JPEG, JPEG2000, BPG and the deep learning based method are presented.