Documents
Poster
MULTI-DOMAIN UNPAIRED ULTRASOUND IMAGE ARTIFACT REMOVAL USING A SINGLE CONVOLUTIONAL NEURAL NETWORK
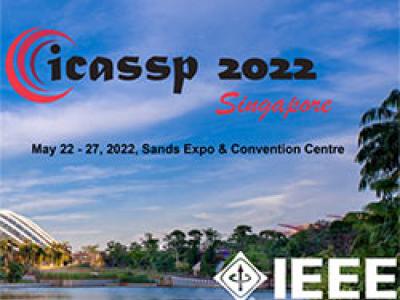
- Citation Author(s):
- Submitted by:
- Jaeyoung Huh
- Last updated:
- 6 May 2022 - 12:41am
- Document Type:
- Poster
- Document Year:
- 2022
- Event:
- Categories:
- Log in to post comments
Ultrasound imaging (US) often suffers from distinct image artifacts from various sources. Classic approaches for solving these problems are usually model-based iterative approaches that have been developed specifically for each type of artifact, which are often computationally intensive. Recently, deep learning approaches have been proposed as computationally efficient and high performance alternatives. Unfortunately, in the current deep learning approaches, a dedicated neural network should be trained with matched training data for each artifact type. This poses a fundamental limitation in the practical use of deep learning for US, since large number of paired data is required for supervised training of multiple models to deal with various US image artifacts. Inspired by the recent success of multi-domain image transfer, herein, we propose a novel unpaired deep learning approach where a single neural network can deal with different types of US artifacts simply by changing a mask vector that switches between different target domains. The proposed method can generate high quality images by removing distinct artifacts, which are comparable to those obtained by separately trained multiple neural networks.