Documents
Presentation Slides
Multi-Label Classification for Automatic Human Blastocyst Grading with Severely Imbalanced Data
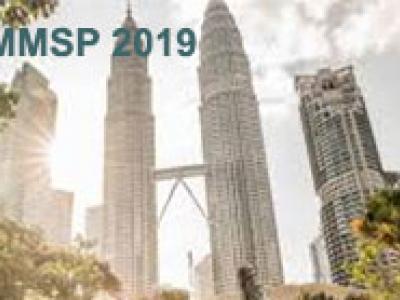
- Citation Author(s):
- Submitted by:
- Lisette Lockhart
- Last updated:
- 24 September 2019 - 6:18pm
- Document Type:
- Presentation Slides
- Document Year:
- 2019
- Event:
- Presenters:
- Lisette Lockhart
- Paper Code:
- MMSP-167
- Categories:
- Log in to post comments
Quality scores assigned to blastocyst inner cell mass (ICM), trophectoderm (TE), and zona pellucida (ZP) are critical markers for predicting implantation potential of a human blastocyst in IVF treatment. Deep Convolutional Neural Networks (CNNs) have shown success in various image classification tasks, including classification of blastocysts into two quality categories. However, the problem of multi-label multi-class classification for blastocyst grading remains unsolved. In this paper, a single CNN is proposed to automatically classify human microscopic blastocyst images in three grading labels, each with multiple quality classes. Additionally, network training with severely imbalanced data (i.e. minority class representing <5% of total samples) is addressed using parameter sharing and stratified sampling schemes. Network parameters associated with each output label are trained jointly, achieving accuracy of 73.9% for ICM grading, 67.3% for TE grading, and 81.8% for ZP grading. This represents accuracy improvement of 3.1%, 18.3%, and 18.2% for ICM, TE, and ZP grading, respectively, over three baseline models.