Documents
Presentation Slides
Multi-level deep neural network adaptation for speaker verification using MMD and consistency regularization
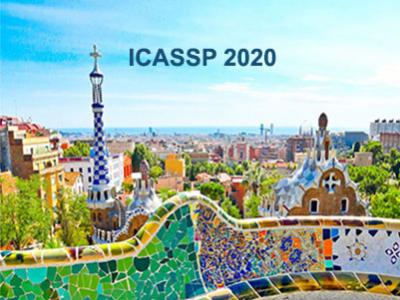
- Citation Author(s):
- Submitted by:
- Man-Wai Mak
- Last updated:
- 13 May 2020 - 10:05pm
- Document Type:
- Presentation Slides
- Document Year:
- 2020
- Event:
- Presenters:
- Man-Wai MAK
- Paper Code:
- 3043
- Categories:
- Log in to post comments
Adapting speaker verification (SV) systems to a new environ- ment is a very challenging task. Current adaptation methods in SV mainly focus on the backend, i.e, adaptation is carried out after the speaker embeddings have been created. In this paper, we present a DNN-based adaptation method using maximum mean discrepancy (MMD). Our method exploits two important aspects neglected by previous research. First, instead of minimizing domain discrepancy at utterance-level alone, our method minimizes domain discrepancy at both frame-level and utterance-level, which we believe will make the adaptation more robust to the duration discrepancy be- tween training data and test data. Second, we introduce a consistency regularization for unlabelled target-domain data. The consistency regularization encourages the target speaker embeddings robust to adverse perturbations. Experiments on NIST SRE 2016 and 2018 show that our DNN adaptation works significantly better than the previously proposed DNN adaptation methods. What’s more, our method works well with backend adaptation. By combining the proposed method with backend adaptation, we achieve a 9% improvement over backend adaptation in SRE18.