Documents
Poster
Multi-Task Learning in Autonomous Driving Scenarios Via Adaptive Feature Refinement Networks
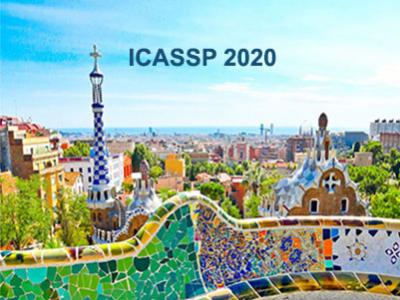
- Citation Author(s):
- Submitted by:
- Mingliang Zhai
- Last updated:
- 14 May 2020 - 4:43am
- Document Type:
- Poster
- Document Year:
- 2020
- Event:
- Presenters:
- Mingliang Zhai
- Paper Code:
- 1406
- Categories:
- Log in to post comments
Many deep learning applications benefit from multi-task learning with several related objectives. In autonomous driving scenarios, being able to accurately infer motion and spatial information is essential for scene understanding. In this paper, we combine an adaptive feature refinement module and a unified framework for joint learning of optical flow, depth and camera pose estimation in an unsupervised manner. The feature refinement module is embedded into motion estimation and depth prediction sub-networks, which can exploit more channel-wise relationships and contextual information for feature learning. Given a monocular video, our network firstly estimates depth and camera motion, and calculates rigid optical flow. Then, we design an auxiliary flow network for inferring non-rigid flow fields. In addition, a
forward-backward consistency check is adopted for occlusion reasoning. Extensive experiments on KITTI dataset demonstrate that the proposed method achieves potential results comparing to recent deep learning networks.