Documents
Presentation Slides
MULTI-TASK LEARNING WITH COMPRESSIBLE FEATURES FOR COLLABORATIVE INTELLIGENCE
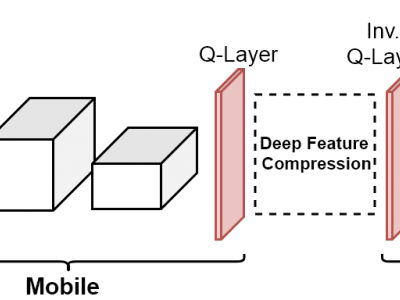
- Citation Author(s):
- Submitted by:
- Saeed Ranjbar Alvar
- Last updated:
- 20 September 2019 - 2:18pm
- Document Type:
- Presentation Slides
- Document Year:
- 2019
- Event:
- Presenters:
- Saeed Ranjbar Alvar
- Paper Code:
- 3572
- Categories:
- Log in to post comments
A promising way to deploy Artificial Intelligence (AI)-based services on mobile devices is to run a part of the AI model (a deep neural network) on the mobile itself, and the rest in the cloud. This is sometimes referred to as collaborative intelligence. In this framework, intermediate features from the deep network need to be transmitted to the cloud for further processing. We study the case where such features are used for multiple purposes in the cloud (multi-tasking) and where they need to be compressible in order to allow efficient transmission to the cloud. To this end, we introduce a new loss function that encourages feature compressibility while improving system performance on multiple tasks. Experimental results show that with the compression-friendly loss, one can achieve around 20% bitrate reduction without sacrificing the performance on several vision-related tasks.
ICIP_2019.pptx
