Documents
Presentation Slides
Presentation Slides
MULTICHANNEL SPEECH SEPARATION WITH RECURRENT NEURAL NETWORKS FROM HIGH-ORDER AMBISONICS RECORDINGS
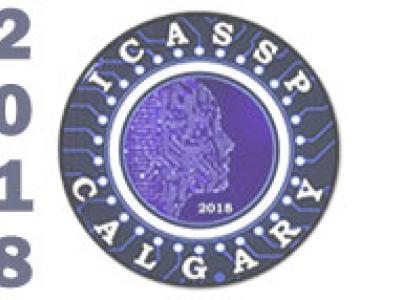
- Citation Author(s):
- Submitted by:
- Laureline Perotin
- Last updated:
- 19 April 2018 - 5:18pm
- Document Type:
- Presentation Slides
- Document Year:
- 2018
- Event:
- Presenters:
- Laureline Perotin
- Paper Code:
- AASP-L2.2
- Categories:
- Log in to post comments
We present a source separation system for high-order ambisonics (HOA) contents. We derive a multichannel spatial filter from a mask estimated by a long short-term memory (LSTM) recurrent neural network. We combine one channel of the mixture with the outputs of basic HOA beamformers as inputs to the LSTM, assuming that we know the directions of arrival of the directional sources. In our experiments, the speech of interest can be corrupted either by diffuse noise or by an equally loud competing speaker. We show that adding as input the output of the beamformer steered toward the competing speech in addition to that of the beamformer steered toward the target speech brings significant improvements in terms of word error rate.
perotin.pdf
