Documents
Poster
Multiple-Graph Recurrent Graph Convolutional Neural Network architectures for predicting disease outcomes
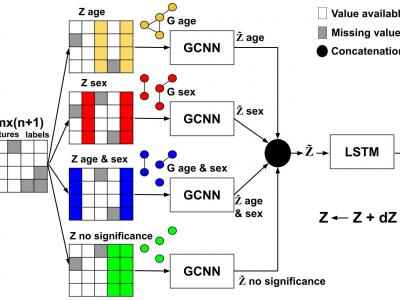
- Citation Author(s):
- Submitted by:
- Juliette Valenchon
- Last updated:
- 11 May 2019 - 1:04pm
- Document Type:
- Poster
- Document Year:
- 2019
- Event:
- Presenters:
- Juliette Valenchon
- Paper Code:
- 3600
- Categories:
- Log in to post comments
Improving disease outcome prediction can greatly aid in the strategic deployment of secondary prevention approaches. We develop a method to predict the evolution of diseases by taking into account personal attributes of the subjects and their relationships with medical examination results. Our approach builds upon a recent formulation of this problem as a graph-based geometric matrix completion task. The primary innovation is the introduction of multiple graphs, each relying on a different combination of subject attributes. Via statistical significance tests, we determine the relevant graph(s) for each medically-derived feature. We then employ a multiple-graph recurrent graph convolutional neural network architecture to predict the disease outcomes. We demonstrate the efficacy of the technique by addressing the task of predicting the development of Alzheimer’s disease for patients exhibiting mild cognitive impairment, showing that the incorporation of multiple graphs improves predictive capability.
Link to the paper: https://ieeexplore.ieee.org/document/8683433