Documents
Poster
NEWS RECOMMENDATION VIA MULTI-INTEREST NEWS SEQUENCE MODELLING
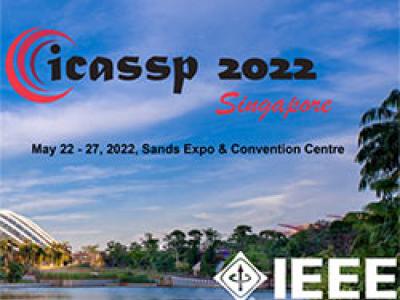
- Citation Author(s):
- Submitted by:
- Wenpeng Lu
- Last updated:
- 6 May 2022 - 2:44am
- Document Type:
- Poster
- Document Year:
- 2022
- Event:
- Presenters:
- Rongyao Wang
- Paper Code:
- SPE-67.4, ICASSP 5690
- Categories:
- Keywords:
- Log in to post comments
A session-based news recommender system recommends the next news to a user by modeling the potential interests embedded in a sequence of news read/clicked by her/him in a session. Generally, a user's interests are diverse, namely there are multiple interests corresponding to different types of news, e.g., news of distinct topics, within a session. However, most of existing methods typically overlook such important characteristic and thus fail to distinguish and model the potential multiple interests of a user, impeding accurate recommendation of the next piece of news. Therefore, this paper proposes multi-interest news sequence (MINS) model for news recommendation. In MINS, a news encoder based on self-attention is devised on learn an informative embedding for each piece of news, and then a novel parallel interest network is devised to extract the potential multiple interests embedded in the news sequence in preparation for the subsequent next-news recommendations. The experimental results on a real-world dataset demonstrate that our model can achieve better performance than the state-of-the-art compared models. Our source code is publicly available on GitHub.