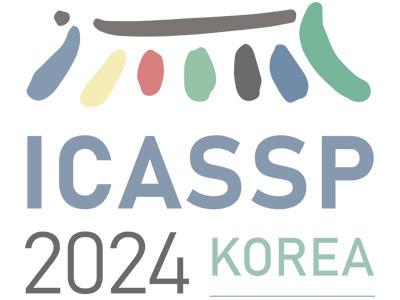
- Read more about MDRT: MULTI-DOMAIN SYNTHETIC SPEECH LOCALIZATION
- Log in to post comments
With recent advancements in generating synthetic speech, tools to generate high-quality synthetic speech impersonating any human speaker are easily available. Several incidents report misuse of high-quality synthetic speech for spreading misinformation and for large-scale financial frauds. Many methods have been proposed for detecting synthetic speech; however, there is limited work on localizing the synthetic segments within the speech signal. In this work, our goal is to localize the synthetic speech segments in a partially synthetic speech signal.
mdrt_v05.pdf

- Categories:
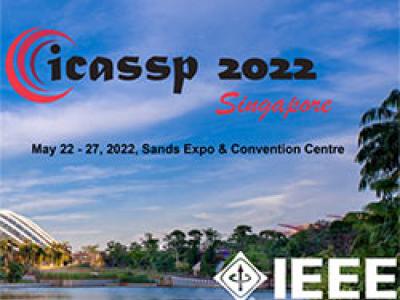
- Read more about Entrainment Analysis for Assessment of Autistic Speech Prosody Using Bottleneck Features of Deep Neural Network
- Log in to post comments
In the present study, we quantify entrainment characteristics of conversation with the aim of automatic assessment of the severity of autism spectrum disorder (ASD). We focus on pairs of utterances immediate before and after turn-takings, which have prosodic/acoustic similarities.
- Categories:
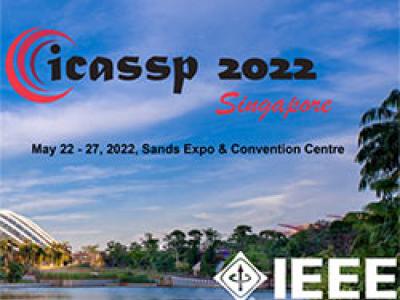
- Read more about NEWS RECOMMENDATION VIA MULTI-INTEREST NEWS SEQUENCE MODELLING
- Log in to post comments
A session-based news recommender system recommends the next news to a user by modeling the potential interests embedded in a sequence of news read/clicked by her/him in a session. Generally, a user's interests are diverse, namely there are multiple interests corresponding to different types of news, e.g., news of distinct topics, within a session. However, most of existing methods typically overlook such important characteristic and thus fail to distinguish and model the potential multiple interests of a user, impeding accurate recommendation of the next piece of news.
- Categories: