Documents
Presentation Slides
Noise-Disentangled Graph Contrastive Learning via Low-Rank and Sparse Subspace Decomposition
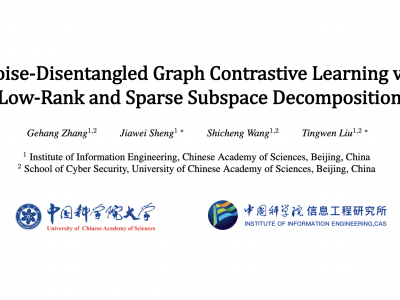
- DOI:
- 10.60864/9rfd-b084
- Citation Author(s):
- Submitted by:
- Gehang Zhang
- Last updated:
- 6 June 2024 - 10:27am
- Document Type:
- Presentation Slides
- Document Year:
- 2024
- Event:
- Presenters:
- Gehang Zhang
- Paper Code:
- MLSP-L18
- Categories:
- Log in to post comments
Graph contrastive learning aims to learn a representative model by maximizing the agreement between different views of the same graph. Existing studies usually allow multifarious noise in data augmentation, and suffer from trivial and inconsistent generation of graph views. Moreover, they mostly impose contrastive constraints on pairwise representations, limiting the structural correlations among multiple nodes. Both problems may hinder graph contrastive learning, leading to suboptimal node representations. To this end, we propose a novel graph contrastive learning framework, namely GCL-LS, via low-rank and sparse subspace decomposition. In particular, it decomposes node representations into low-rank and sparse components, preserving structural correlations and compressed features in the low-rank and sparse subspace, respectively. By contrasting the representations in the subspaces, it naturally disentangles low-quality noise in data augmentation, and captures structural correlations and substantial features of nodes in contrastive learning. Experimental results show that our method significantly improves downstream node classification accuracy, and further analysis demonstrates the effectiveness of the subspace decomposition in graph contrastive learning.