Documents
Poster
Noisy-ArcMix: Additive Noisy Angular Margin Loss Combined With Mixup for Anomalous Sound Detection
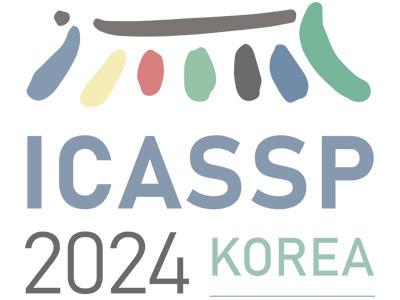
- DOI:
- 10.60864/7a8t-9263
- Citation Author(s):
- Submitted by:
- Soonhyeon Choi
- Last updated:
- 6 June 2024 - 10:32am
- Document Type:
- Poster
- Document Year:
- 2024
- Event:
- Presenters:
- Soonhyeon Choi
- Paper Code:
- AASP-P10.6
- Categories:
- Log in to post comments
Unsupervised anomalous sound detection (ASD) aims to identify anomalous sounds by learning the features of normal operational sounds and sensing their deviations. Recent approaches have focused on the self-supervised task utilizing the classification of normal data, and advanced models have shown that securing representation space for anomalous data is important through representation learning yielding compact intra-class and well-separated intra-class distributions. However, we show that conventional approaches often fail to ensure sufficient intra-class compactness and exhibit angular disparity between samples and their corresponding centers. In this paper, we propose a training technique aimed at ensuring intra-class compactness and increasing the angle gap between normal and anomalous samples. Furthermore, we present an architecture that extracts features for important temporal regions, enabling the model to learn which time frames should be emphasized or suppressed. Experimental results demonstrate that the proposed method achieves the best performance giving 0.90%, 0.83%, and 2.16% improvement in terms of AUC, pAUC, and mAUC, respectively, compared to the state-of-the-art method on DCASE 2020 Challenge Task2 dataset.