Documents
Presentation Slides
Non-separable Wavelet Transform Using Learnable Convolutional Lifting Steps
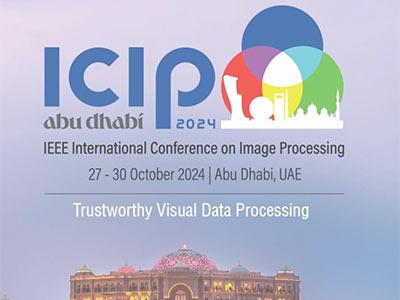
- DOI:
- 10.60864/2ysj-hw71
- Citation Author(s):
- Submitted by:
- Joao Parracho
- Last updated:
- 8 November 2024 - 9:53am
- Document Type:
- Presentation Slides
- Document Year:
- 2024
- Event:
- Presenters:
- João Oliveira Parracho
- Paper Code:
- MA1.L2.3
- Categories:
- Log in to post comments
Wavelet transforms have been a relevant topic in signal processing for many years. One of the most common strategies when designing wavelet transforms is the use of lifting schemes, known for their perfect reconstruction properties and flexible design. This paper introduces a novel 2D non-separable lifting design methodology based on deep learning architectures. The proposed method is assessed within the context of end-to-end lossless image compression. The attained results highlight a significant 99% reduction of the number of learnable coefficients, with a direct impact on the computational complexity, while still achieving higher compression performance when compared to other end-to-end state-of-the-art wavelet-based frameworks. The code for the models used to obtain the reported results are available at https://github.com/joaoparracho/2D-NSWT-LCLS.