Documents
Presentation Slides
Presentation Slides
NORMALIZED LEAST-MEAN-SQUARE ALGORITHMS WITH MINIMAX CONCAVE PENALTY
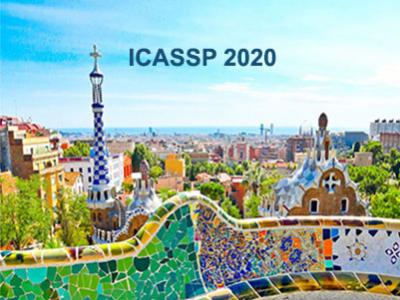
- Citation Author(s):
- Submitted by:
- Hiroyuki Kaneko
- Last updated:
- 15 May 2020 - 8:00pm
- Document Type:
- Presentation Slides
- Document Year:
- 2020
- Event:
- Presenters:
- Hiroyuki Kaneko
- Paper Code:
- SPTM-L6.2
- Categories:
- Log in to post comments
We propose a novel problem formulation for sparsity-aware adaptive filtering based on the nonconvex minimax concave (MC) penalty, aiming to obtain a sparse solution with small estimation bias. We present two algorithms: the first algorithm uses a single firm-shrinkage operation, while the second one uses double soft-shrinkage operations. The twin soft-shrinkage operations compensate each other, promoting sparsity while avoiding a serious increase of biases. The whole cost function is convex in certain parameter settings, while the instantaneous cost function is always nonconvex. Numerical examples show the superiority compared to the existing sparsity-aware adaptive filtering algorithms in system mismatch and sparseness of the solution.